Protect your operations with digital twin predictive maintenance
There’s nothing more costly than an unexpected breakdown. That’s why effective scheduling and repairs are essential. Digital twin predictive maintenance ensures accurate, effective, and timely refurbishment of machinery and processes that feed your production line.
What is predictive maintenance?
Simply put, predictive maintenance is fixing things that aren’t broken yet to avoid serious consequences that can impact your business. Of course, this isn’t a new concept for most industries. Most business operations have repair and maintenance schedules.
Machines and industrial equipment will inevitably get damaged or deteriorate from environmental conditions, use, and time. As such, repairing broken components is an expected part of most companies’ expected expenses. However, the real cost incurred by businesses comes from forced downtime due to malfunctioning machinery.
Predictive maintenance helps get ahead of these big breakdowns and helps avoid downtime. Proactively performing maintenance and care on machinery also ensures safety standards are met, and problems are detected before they snowball out of control.
Leaving traditional predictive maintenance behind
Data helps make decisions – but not all data is equal, and not every algorithm is designed to keep up with changing environmental conditions. Traditional predictive maintenance is usually built on data which might not integrate real-time information or the context of external factors.
Different components in a system may get swapped out. Environments change. Static ata originally used to build a maintenance schedule grows outdated. Inaccurate data leads to ineffective data utilisation.
However, collecting high-fidelity data is only part of the problem. The algorithms you use must be capable of quickly adjusting to new parameters, or you risk inaccurate calculations or predictions. Without the real-time, holistic approach of digital twin tech, predictive maintenance can be like glimpsing only the ears of an elephant – but never the whole animal.
Using a digital twin for predictive maintenance
With digital twin predictive maintenance, engineers can visualise and study your most critical product systems in a 3D virtual model, within the context of the same real-life environmental conditions.
What is a digital twin? A digital twin is a virtual representation of a real-life asset, process, or system that continuously communicates with its physical counterpart.
Digital twin maintenance solutions promptly identify irregularities, including cracks, deformations, and other problems. Algorithms transform complex silos of data into a visual inventory which can accurately predict when a component needs to be fixed or replaced, based on their health and performance.
The benefits of digital twin predictive maintenance
Digital twin maintenance protocols empower you to make proactive, data-driven decisions. This saves you valuable time and money, while extending asset lifespan. Schedules based on time alone can result in spending too much on checks you don’t need – or missing the early signs of an impending breakdown. Companies can mitigate overservicing and assign repairs when and where you actually need them.
Furthermore, digital twins allow multiple experts and stakeholders to work together via the virtual replica to build predictive models and solve problems remotely. This both saves on expensive flights, and provides each team member a birdseye view of the issue.
Proactive solutions overtake preventative actions
Predictive digital twin solutions go beyond preventing structural failures. Effective and efficient maintenance also leads to optimised energy consumption, better response management, and leaner operations.
Engineers using digital twin predictive modelling avoid the shortcomings of trial-and-error solutions. Your team can experiment with adjustments, upgrades, and suggested changes without physically affecting the asset, which you can find the best modification before implementing them in real life.
Integrating digital twin predictive maintenance
Most machinery, systems, and supply chains already have a solid digital footprint. Manufacturers have stacks of CAD drawings, diagnostic data, and more digital information. Yet so many companies lose key insights in the depths of data silos.
To model, verify, and deploy a digital twin, a company needs to connect smart sensors to multiple functions and platforms, while obtaining the computing capacity to process all the data. While you may have legacy tools available, you might require more platforms, software, and digitisation to reach digital maturity.
If you’re unsure of how to integrate digital twin predictive maintenance in your business operations, the team at Draga are ready to help. There’s no project too complex for us to handle, and no industry we won’t explore. Get in touch today and let us help your business think.
You May Also Like
These Related Stories
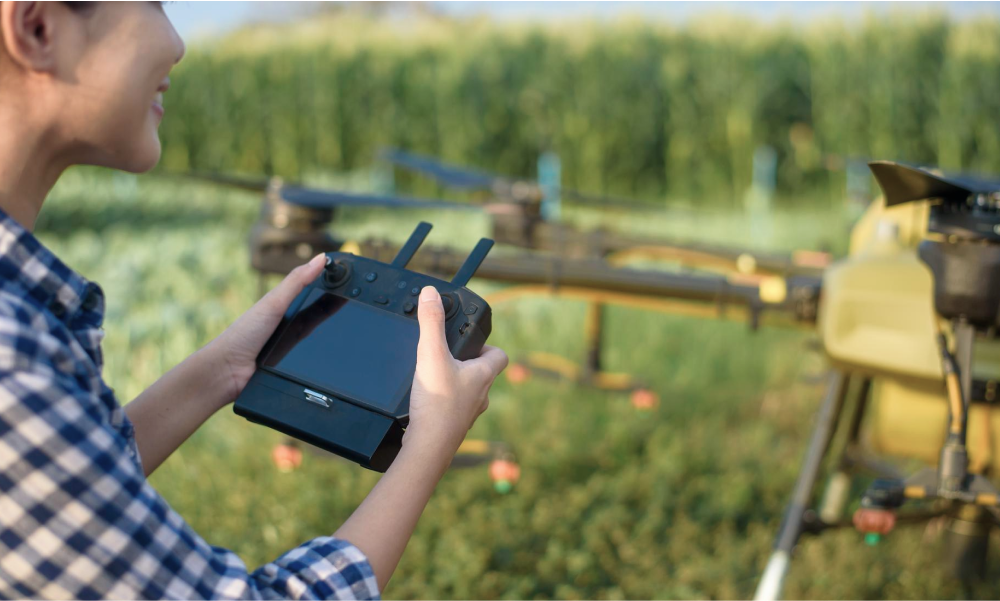
Why is engineering digital twin technology important?
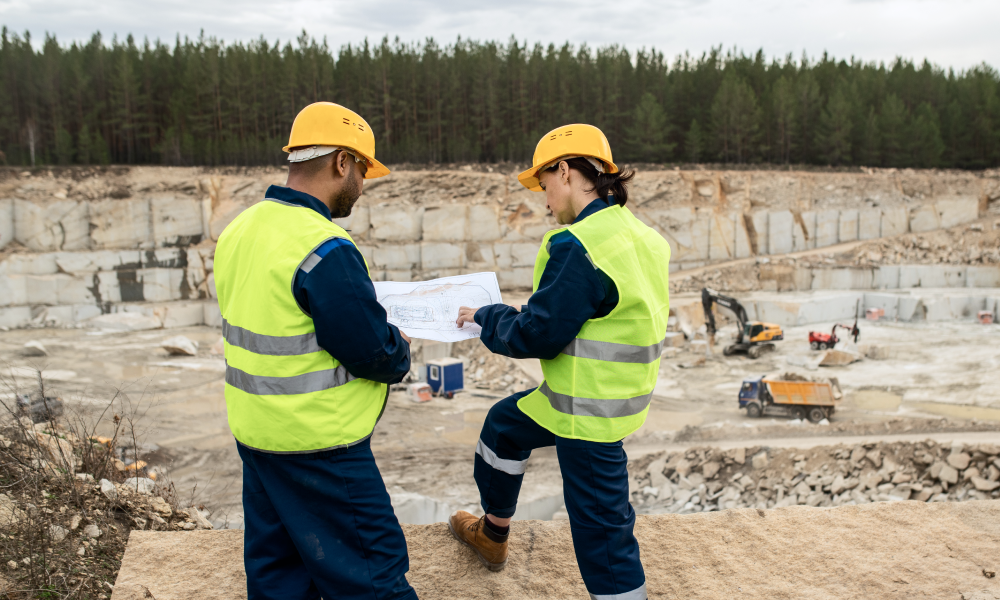
Overlooked challenges in digital twin
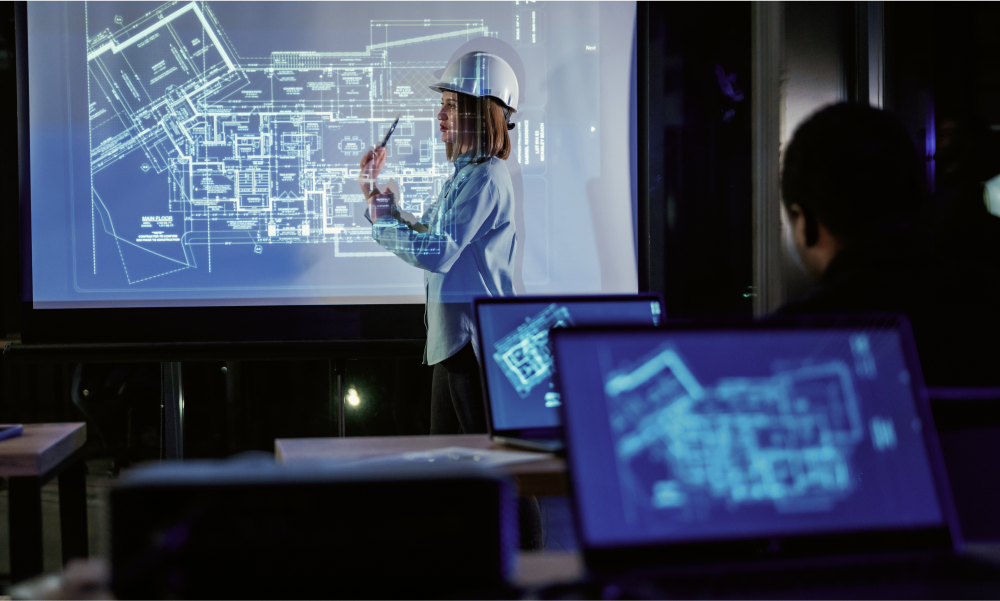